Picture a car behind the wheel, swerving past oncoming traffic, braking for pedestrians and obeying traffic signals, all on its own. Or consider doctors identifying small signs of disease in medical scans before they become serious. AI in computer vision makes possible such amazing feats. It allows machines to “see” and comprehend images the way we do.
Computer vision AI is on fast track. It is altering the way that many businesses operate. This piece covers the building blocks of computer vision AI. You’ll find out how it works and where it’s used, as well as what to expect going forward.
A Guide to General Concepts in Computer Vision
Computer vision is not simply showing a computer a picture. It’s about teaching it to know what it sees. This section explains the steps to make it happen and the tech that ends up behind the scenes.
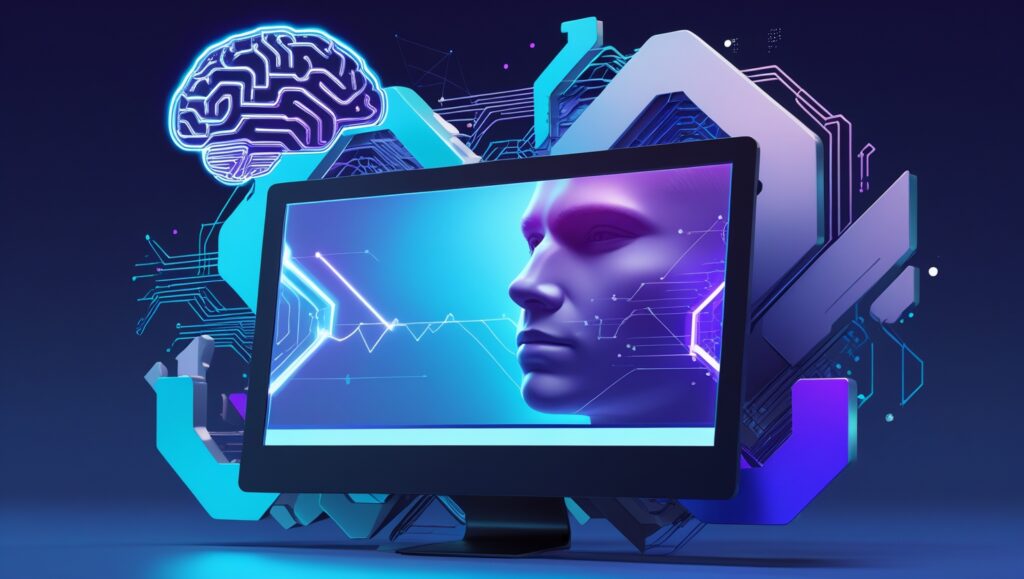
Step-by-Step Guide on How Computer Vision Works
There are a few basic steps that computer vision contains. Images are first captured using cameras. Then, they’re prepared to facilitate analysis. It’s like cleaning up a photo before sharing it. Then, the computer extracts key details. Lastly, it uses those details to identify what’s in the visual.
Digital images, unlike traditional photographs, are composed of small blocks known as pixels. Each pixel has a color. These colors are numbers in their eyes. Computer vision means making sense of the contents of the image from those numbers.
Deep Learning for computer vision
Many tools are available to enable “vision” for computers. One key approach employs Convolutional Neural Networks (CNNs). CNNs excel at detecting trends in images. They are used, for example, to identify objects.
Examples: Models for object detection (finding specific objects in an image) such as YOLO, Faster R-CNN We will be using a type of pre-processing called image segmentation which divides an image into its components. This allows computers to have a better understanding of the scene.
This work has lit the fire within the deep learning community.
Computer vision has been greatly accelerated by deep learning. It enables machines to learn from large amounts of data. It can solve visual tasks. Deep learning outperforms classical methods because it learn relevant features from the images automatically. This means it requires less human intervention.
Practical Implementations of Computer Vision AI
Computer Vision AI is shaking up a number of industries. It’s assisting doctors, streamlining factories and simplifying shopping. Now, let’s turn to some particular examples.
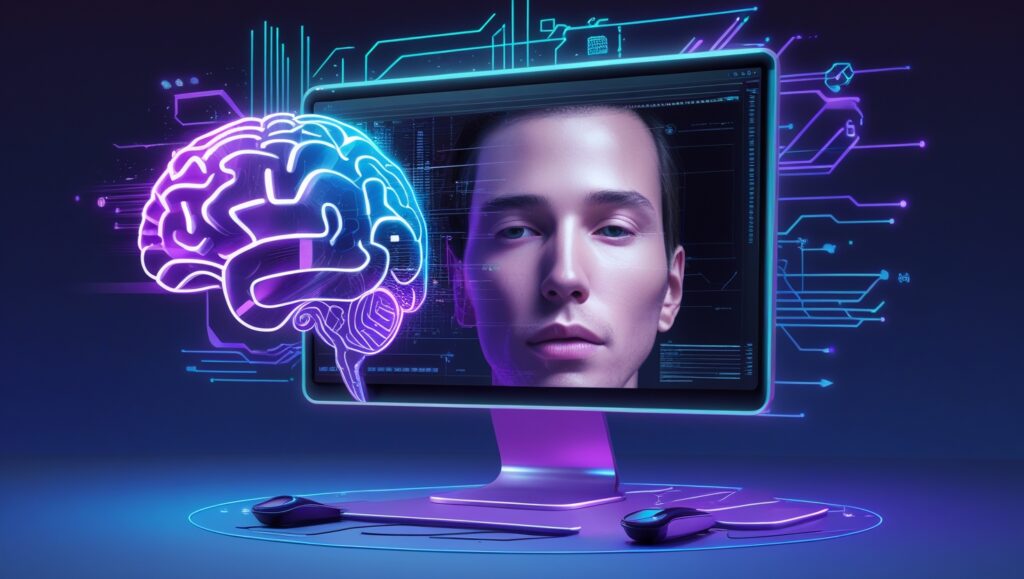
Computer Vision in Healthcare: Improving Diagnostics and Treatment
In healthcare, computer vision AI examines medical images such as X-rays and MRIs. It can also identify diseases and other problems faster and more accurately than humans can on their own. It can even assist surgeons in operating rooms by providing a clearer picture of the body.
Machine Vision for Manufacturing Quality Control & Automation
INSPECTING FOR DEFECTS AT FACTORIES WITH COMPUTER VISION AI This ensures that only quality products reach the customers. It can guide robots. Which makes production fast and reliable. It can even forecast when machines require maintenance.
Computer Vision and Its Hype in the Retail Experience
The primary applications of computer vision AI for retailers It is used by self-checkout systems to recognize items. This accelerates the checkout experience. It can also offer recommendations. This is predicated on what they have purchased in the past. Computer vision can also be used for inventory tracking. This ensures that shelves never run out.
Computer Vision AI CAPTURES CHALLENGES
As powerful; as computer vision AI is, it has its limitations. It needs lots of data. It is also very computationally expensive. These barriers will need to be overcome before widespread use.
Computer Vision 101 — Part 5: Data Requirements and Bias
At that time, computer vision models were very data-hungry. It requires high-quality data that is free from bias. TRAINING ↠ The working hypothesis is: If we have biased data, then the model will be biased as well. For example, if a facial recognition system is trained on images of a single race, people of other races may not be accurately recognized with the model.
Computational Costs and Infrastructure Requirements
Computer vision models tend to require quite a bit of computing power to run and train. That generally involves using specialized hardware, such as GPUs. So it means using cloud services. This can be costly, particularly with large models.
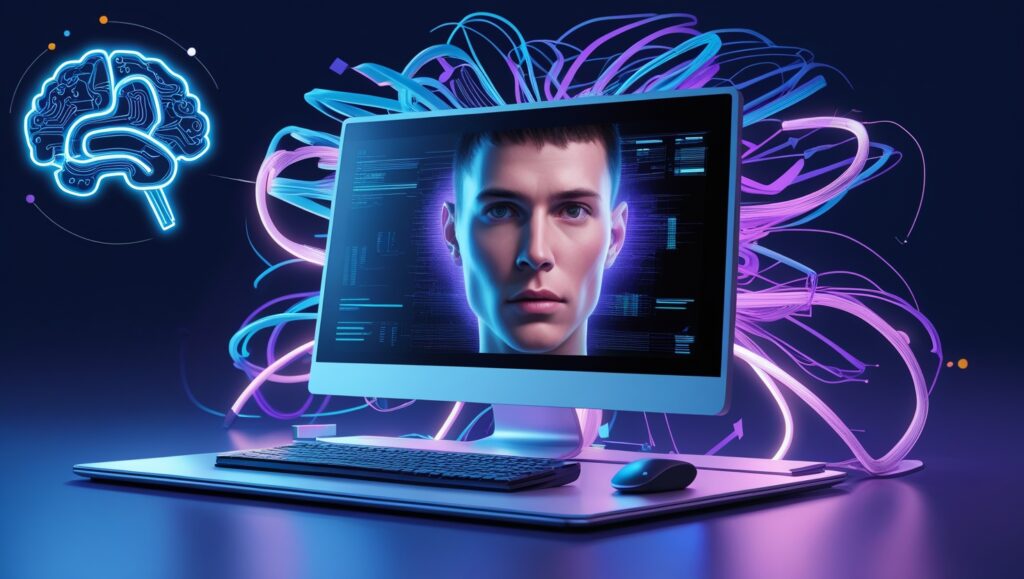
Ethical Challenges and Privacy Issues
Ethical Issues of Computer Vision Facial recognition and surveillance are clear uses. Also, it is important to protect privacy. You also want to make sure that you’re using the technology responsibly.”
Where the World of Computer Vision AI is Headed
It is also becoming stale as the computer vision AI undergoes constant change. We can expect several things such as new AI techniques, edge computing, and new applications to arrive in the near future. The future looks promising.
AI and Machine Learning Advancements
Transformers, generative models and other breakthroughs can improve computer vision further. Transformers make it possible for computers to understand the context in the images. Generative models generate images on the base of existing images.
Machine Vision–Era of Edge Computing and RealTime Computer Vision
Edge computing allows computer vision to move closer to where the data is generated. This means running images through sets of programs on devices. These devices consist of phones, cameras, and more. This reduces delays. It can help to inspire real-time applications. Imagine self-driving vehicles instantly responding to those ever-changing road conditions.
Computer vision applications have evolved over time
AI computer vision will probably expand to new industry areas We will likely see it more used in agriculture, environmental monitoring, and security. The options are virtually
limitless.
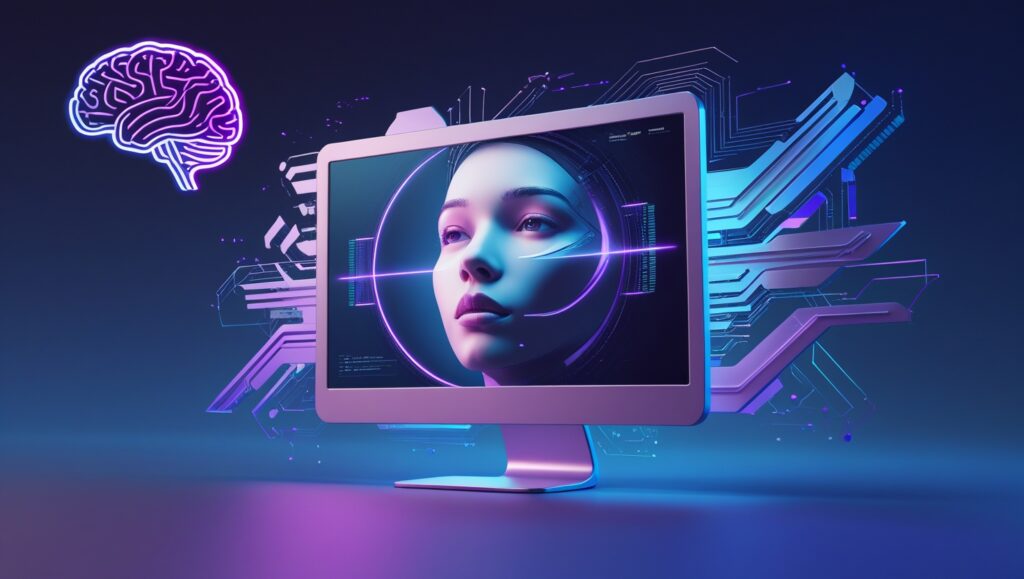
Conclusion
So far, computer vision AI is an amazing tool. It’s transforming how machines visualize and comprehend the world. It benefits industrial sectors. This technology will transform our lives in innumerable ways. Want to know more? You are familiar with data until October 2023.